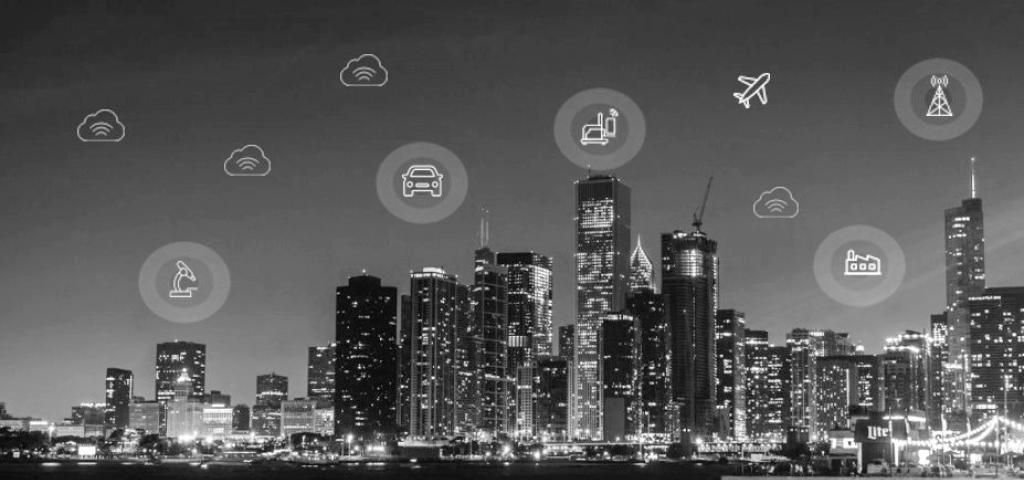
The Intelligent Edge: A New Frontier for AI Innovation
AI systems have evolved. Over the past few years, they have moved far beyond data analysis and real-time insights, now accessing vast amounts of structured and unstructured content to generate new ideas, solve complex problems, and suggest practical actions. Generative AI, powered by large language models (LLMs) and advanced frameworks, is unlocking unprecedented possibilities.
AI is primarily trained, built, deployed, and operated in the cloud. That’s because current-generation AI demands extensive computational power, storage, and networking. It relies on specialized chips that are available only in large data centers that require reliable power and water resources.
However, while AI is developed in the cloud, its full potential will ultimately be unlocked at the edge, where people will directly experience and interact with AI-driven technologies.
Early industry examples of edge-based AI include facial and fingerprint recognition on mobile devices and autonomous systems such as robots, drones, autonomous vehicles, and surveillance systems.
In the years ahead, the intelligent edge will democratize AI adoption and inference across industries, inspiring as-yet-unimagined applications. Success requires a robust platform that is secure, high performing, and efficient in both power and computational ability.
Honing the intelligent edge
The current generation of embedded and edge systems serves industries such as aerospace, defense, industrial, medical, automotive, and telecommunications. Much of these systems’ application logic relies on statically compiled code or dynamically loaded libraries, with minimal embedded intelligence. IoT technologies allow basic telemetry data from these devices to be collected, stored, and analyzed in the cloud. The resulting dashboard-driven descriptive analytics and machine learning-based predictive analytics have made a real difference for those industries and the humans who depend on them.
But that transformation is just the beginning. With the integration of diverse sensors into devices and rapid advancements in nanoscale chip technology, significantly more data can be captured and processed directly at the edge, without needing to route to the cloud. Additionally, silicon manufacturers are embedding AI capabilities within systems-on-chip (SoCs), which allows compact AI runtimes and enables frameworks to run on a variety of processors, including MPUs, MCUs, NPUs, CPUs, GPUs, FPGAs, and ASICs. These capabilities span silicon architectures such as x86, Arm, and RISC-V. They require real-time operating systems and Linux, which are predominant in embedded and edge systems, to support AI at the edge.
We expect the intelligent edge to support diverse silicon and the applications that run on it. That means new generations of distributed computing environments must be optimized for power and compute efficiency, compact AI runtimes and frameworks, hardware virtualization, application containerization, and DevSecOps and AIOps for AI-enabled applications.
Building the intelligent edge will create plenty of business benefits, such as:
- Real-time decision-making: Devices can analyze data in real time, which is essential in sectors where split-second decisions are crucial.
- Lower latency: Processing data closer to its source makes it possible to reduce the time to send information to and from centralized cloud platforms.
- Improved security and privacy: When sensitive data is processed locally at the edge, it doesn’t have to be transferred to the cloud, lowering the risk of data breaches.
- Bandwidth efficiency: As the number of connected, intelligent devices grows, the network bandwidth strain is reduced; massive amounts of raw data do not need to be sent across the network to the cloud.
- AI-driven insights: With AI and machine learning (ML) capabilities integrated at the edge, devices can autonomously learn and adapt, gradually improving performance.
Today, the intelligent edge is defined by a fragmented, heterogeneous landscape of silicon architectures, semiconductor providers, operating systems, and tools.
To achieve its full potential, the intelligent edge will have to grow and eventually span the edge-to-cloud continuum, as well as the entire lifecycle of AI-powered applications, devices, and systems. Achieving this future requires a seamless edge-to-cloud infrastructure. Together, the intelligent edge and intelligent cloud will enable an AI-powered world where the physical and digital realms merge seamlessly.
It starts with a diverse portfolio that includes the right set of features. Anyone building applications for these environments needs a consistent operating environment across the data plane, control plane, management framework, and security infrastructure.
Wind River® has a comprehensive software portfolio. It includes real-time operating systems, Linux offerings, hypervisors, DevOps services, lifecycle management solutions, and, most recently, eLxr Pro, our enterprise-grade Linux solution. These tools — and the wisdom and experience gathered from our decades in the industry — help our customers and partners harness the power of the intelligent edge to build, operate, and maintain AI-driven applications across diverse industries.
We aren’t stopping there. Wind River is also building an ecosystem and strategic partnerships with cloud hyperscalers, semiconductor partners, IHVs, ISVs, and AI startups. Each of them offers critical technologies that help our customers incorporate the intelligent edge into their plans to unlock new opportunities.
About the author
By Avijit Sinha, Senior Vice President, Strategy and Global Business Development